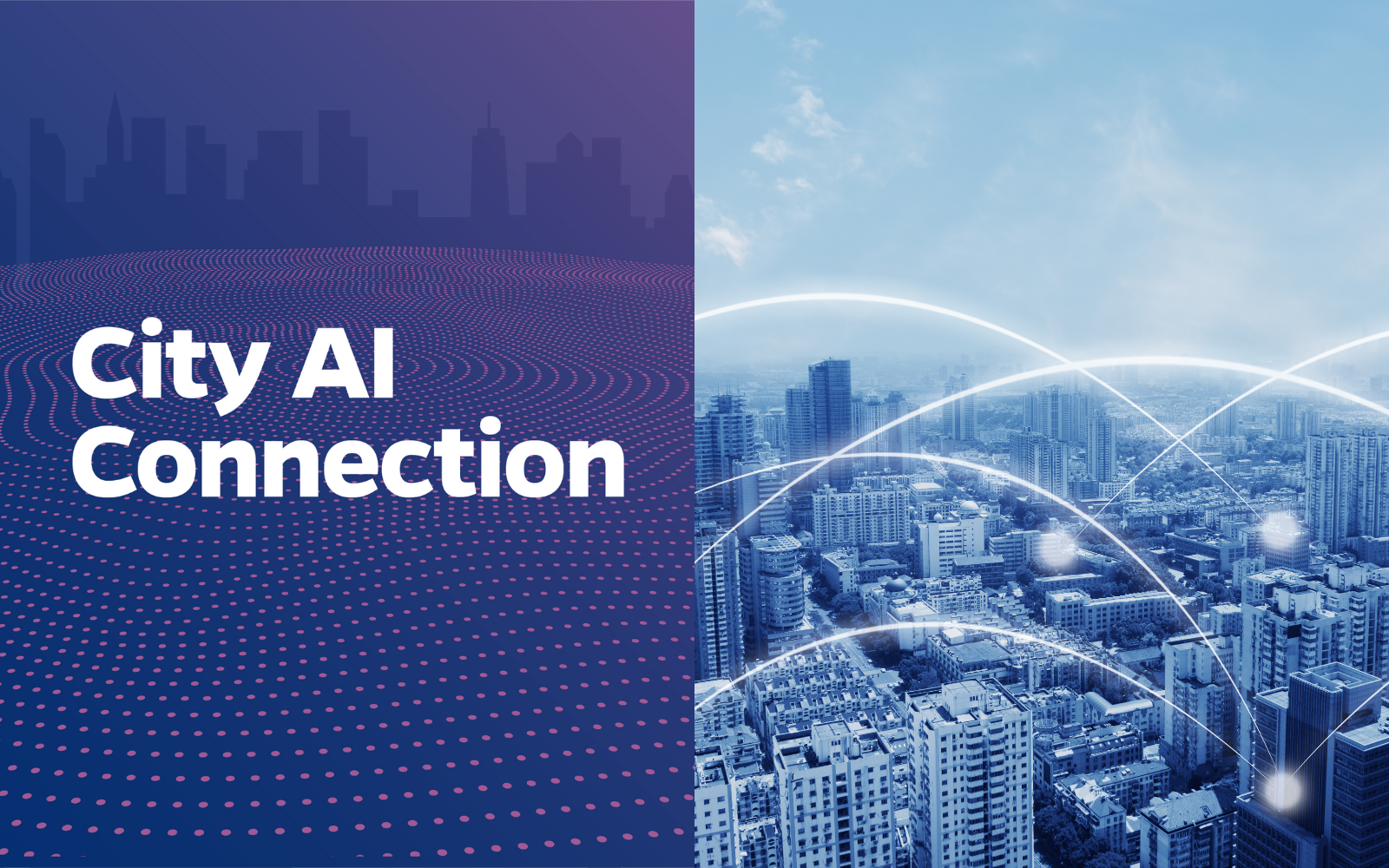
City AI Connection is a series of posts on emerging ideas in artificial intelligence, with a focus on government application. These articles are initially shared exclusively with the City AI Connect community and subsequently with a broader audience via the Bloomberg Center for Government Excellence at Johns Hopkins University website, govex.jhu.edu. City AI Connect is a global learning community and digital platform for cities to trial and advance the usage of generative artificial intelligence to improve public services. The community is open exclusively to local government employees. To join, visit cityaiconnect.jhu.edu.
By Heather Bree and Maeve Mulholland
As governments increasingly adopt predictive models and artificial intelligence in their policies and programs, it is essential they take a values-centered approach to mitigate potential technology-driven inequities like biased decision-making, disproportionate allocation of resources, and negative impacts on marginalized communities.
To do this, public sector leaders can borrow from the concept of Value-Sensitive Design (VSD). Originally described in 1999 by Batya Friedman, VSD is an iterative approach that uses tests, pilots, and evaluations to measure the impact of a technology and assess how well a project upholds predetermined values that promote human wellbeing. Though access to AI tools is relatively nascent, we are already seeing values-driven approaches to government AI strategies. It’s a trend that shows thoughtfulness and a promise to magnify the positive effects of AI tools while reducing the technology’s negative consequences for residents.
To implement a VSD approach, it is imperative project owners define the principles and people an AI project prioritizes. For example, an AI strategy that uses a chat bot to provide information about mental health services might prioritize values like self-efficacy and privacy, and focus on creating equity for residents deterred from seeking help by the stigma surrounding mental health. Implementing an artificial intelligence algorithm will not be perfect, but it is important to remember that a tool does not have to be perfectly fair to be usable. If an algorithm is less biased than an average person, it is an improvement. In fact, “progress, not perfection” is one of the theoretical constructs of VSD.
Most importantly, AI adoption requires a focus on building trust through transparency and accountability by:
- Making all information about the AI being used—including all the analysis and the results of the assessment and evaluation of the tool—easily available to users.
- Launching small beta projects and seeking feedback from impacted communities, incorporating that feedback into future project iterations.
- Investing in thorough training for employees who will use these tools, and providing easy and clear methods for users to report errors and lodge challenges or complaints, which are actively followed up on.
Value Sensitive Design in Practice
A predictive model is intended to support human reasoning, but in practice the model often substitutes for our judgment. This seems to be a driving factor behind mistakes made in law enforcement and the justice system, such as when police in Jacksonville, Florida used facial recognition technology that could not reliably distinguish Black faces as a basis for arrest and prosecution.
However, we are hopeful that thoughtful regulation may combat this problem. In October 2024, Maryland became the first state to dictate when police can use facial recognition software, prohibiting identification through the technology as the sole basis of a prosecution, and requiring police to disclose all uses of facial recognition. Maryland State Sen. Charles Sydnor, who sponsored the new law, points out that police are “not going to stop using [face recognition]. So long as there’s nothing in place, they’re going to continue using it unregulated.”
We can see how value-sensitive design principles may have contributed to this new law. A comparison of the 2022 version of the bill and the final version shows that, among other changes, the legislation was amended to require reporting on the demographics of people identified as potential crime suspects through the use of facial recognition technology. The provision enables analysis of how and when police use this technology, uplifting accountability and transparency values, which independent organizations like the Policing Project at the NYU School of Law advocate for.
“If law enforcement is going to be permitted to use this powerful technology, there must be guardrails in place to provide essential transparency and accountability so that a real assessment of benefits can be made and any harms that might result can be mitigated,” Katie Kinsey, chief of staff to the Policing Project, wrote in the organization’s testimony to the Maryland legislature.
Further, the bill initially only allowed for mugshot and driver identification records to be used as source databases for facial recognition searches, but after an outpouring of pleas from advocates for human trafficking victims, the permissible databases were expanded, with strict regulations, to include other third-party sources, increasing the likelihood of a match and attempting to balance justice for those who have been trafficked with the privacy and safety of those surveilled.
But, even Sydnor points out that the current law is not as strong or comprehensive as it could be, and testimony from the NAACP Legal Defense Fund, Standing Up for Racial Justice Baltimore, and The Innocence Project show there is more room for community input related to equity and racial justice. As other jurisdictions publish their own policies there is opportunity to incorporate this feedback and strengthen protections.
To learn more about incorporating VSD in policy surrounding AI, you can explore the Designing Tech Policy toolkit for instructional case studies and exercises. The VSD Coop wiki also provides a list of other projects related to value sensitive design.
Heather Bree is a data visualization and D3 developer and Maeve Mulholland is a data scientist for GovEx.